Everyone’s seen shows like Black Mirror and read the endless apocalyptic articles about a coming techno-dystopia foisted on society by out-of-control technological development. In recent years, Ted Kaczynski (primarily known for his non-mathematical work) has been rediscovered by radical young primitivists, partly because the consequences of technological advancement are so much more apparent now than when he wrote his manifesto in 1995. Machine learning (ML) in the judicial system has been the staging area for much of this debate, especially because it brings to the surface a host of insecurities, millenarian hopes, and nerd meltdowns, as well as some justified critiques over racial bias, free will, and over-optimization on the priors of criminal behavior.
ML algorithms in the judicial system are increasingly used to predict pre-trial flight risk and appropriate bail rates, as well as assessing a person’s recidivism risk when handing down a prison sentence. But as things stand, ML predictions of the risk of re-offending perform abysmally. The mix of overconfidence and under-performance will wreak havoc on the social fabric. The technology hasn’t reached its goal yet, and it’s unclear when—or even whether—it will do so.
These systems have a lot of problems, from failing to connect with the basic goals of the judicial system, to creating perverse incentives, to falling into self-sabotaging feedback loops, to making the law opaque and unaccountable, to just generally being over- and mis-applied. Ironically, the usual fears of bias in these systems, racial and otherwise, seem to evaporate on examination.
Before getting to the question of whether opaque statistical methods like machine learning are good for the judicial system or not, we should establish the system’s basic goals and purpose.
To start with, the judicial system balances four key purposes:
- Incentives. Prompt, correct, and transparent punishment for specific infractions provides incentives against such infractions for would-be criminals, changing behavior to be less criminal.
- Safety. Convicted criminals, who may be precisely the type to ignore rational incentives, are locked up or executed to prevent further criminal behavior.
- Rehabilitation. Ideally, the punishment of convicted criminals is corrective, in that it changes their pattern of behavior to become lawful, rather than more criminal.
- Orderly Vengeance. The punishment provides closure for victims, offenders, their families and allies, and bystanders, who might otherwise become unsatisfied and take matters into their own hands.
Vengeance isn’t usually regarded as a legitimate purpose of the justice system, but it is in fact a key part of its social role. The desire for vengeance is not irrational; it is the individual instinctual perception of the real need to disincentivize and prevent further criminal behavior. But because everyone may have a different view of the case, unilateral vengeance quickly leads to escalating feuds, as people retaliate against perceived unjust retaliations.
The judicial system is established to act as a trusted neutral party in such disputes, to which everyone can agree to defer. Its standard of what can be proven to a bystander beyond reasonable doubt from a presumption of innocence is designed to eliminate concerns of abuse through false accusations, besides the usual concerns about false convictions. The judicial system is thus a key social technology for coordinating the orderly satisfaction of the rational vengeance instinct. In Girardian terms, effective prevention, deferral, and mitigation of reciprocal mimetic violence is the fundamental foundation of civilized society that allows everything else we consider civilization to exist at all.
This brings us to the question of whether machine learning can improve the judicial system. First, it’s important to consider the question of how ML algorithms affect incentives. The reason to focus on incentives is that crafting correct incentives is generally a harder problem than merely keeping criminals locked up, and even if a ML system could improve the efficiency of short-term safety, doing so at the expense of long-term incentives is counterproductive. So, the question in front of us is: how does ML change the incentives to commit crimes, or otherwise distort behavior?
While the details of these systems are opaque, they generally work on the similar underlying statistical mechanism of finding correlations of features with the variable in question. The system most commonly discussed is COMPAS (Correctional Offender Management Profiling for Alternative Sanctions). An offender fills out a COMPAS questionnaire with 137 questions about geography, age, gender, prior offenses, and more. The system, trained on data from previous years, would issue a score that corresponds to their “risk of re-offending within 2 years.” A judge then uses that score to inform sentencing.
Presumably, features correlated with being a frequent offender, such as number of prior convictions—or living in a high crime area—would point to a higher chance of re-offending. But while both examples could be helpful in predicting the chance of re-offending, these two features are very different from the perspective of incentives. Knowing that the number of priors will be used in the calculus during the sentencing process incentivizes people not to have prior convictions. No problem. But knowledge that living in a particular zip code could drive up a criminal’s sentence, and otherwise make the judicial system consider everyone in the zip code more harshly, incentivizes moving out of that zip code.
While moving out of a particular zip code might be a good choice on a personal level, it is not a good idea to effectively encode this movement in a judicial system. More variables might be added to the ML system, effectively penalizing behavior while bypassing the legislative process. Are you friends with this person? People who are friends with this person have a higher chance of re-offending. Do you listen to this music? This music is correlated with criminality. These incentives might not necessarily be a bad thing, but they are unintended.
For comparison, several publications have raised alarms about a Chinese surveillance system creating a “social score” to control population behavior by assigning points to everyday activities which may themselves not even be criminal. The usage of a “social score” system for everyone in society is, of course, different from usage of a “risk score” system for criminals. However, the underlying algorithmic structure is similar: assign scores and punish people based on a combination of criminal and non-criminal behaviors. It thus raises concerns for many of the same reasons. A major difference is that while the Chinese social score is done deliberately and systematically, machine learning in the judicial system does it accidentally, imprecisely, and in the wrong places.
The fundamental problem is that relying on correlations punishes behaviors correlated with crimes, rather than behaviors that are actually crimes. Even if these correlations are not potentially protected categories, such as age or gender, this is a bad idea, especially if not done deliberately. If you possess a lot of criminal correlates, you may come away with a much harsher sentence, even if you only commit a small offense. This could undermine the judicial system’s ability to deter further crimes and also lead to a sense of injustice that undermines the all-important trust in the system.
Sometimes, a government does want to design an incentive structure to encourage model citizens, like in China’s social credit system. This has its own sticky set of challenges and pitfalls, though it’s more appropriate than trying to jam this set of priorities into the judicial system as such. The probability that a good social engineering policy accidentally happens to fall out as a side effect of a good predictive justice policy is basically nil.
Incentive distortion is not the only concern. These systems are likely to suffer from unchecked and undesirable feedback loops. Let’s say, for example, that the system version 1 has decided that all murderers are too dangerous to release into the general public, and so every murderer is now sent to prison for life. As a result, no murderer commits any new additional crimes. But when it comes time to retrain the system, how should this new version treat the probability of recidivism for a murderer? The data show that no murderer has committed a crime after the initial one; thus, the chance of recidivism is zero. If one filters out the data for where murders occur, then the new version will have no clue what to do when a murder happens. Even if it works, it will likely ignore the crime itself and focus on other factors, which would lead to inaccuracies.
It’s hard to know how well existing systems handle these feedback loops. The training and data cleaning process is not public. Properly making sure that criminals considered dangerous enough to stay in prison for more than two years are correctly considered by the algorithm is tricky, to say the least. It’s unlikely that COMPAS has fully solved it.
This isn’t just a minor issue, either. The legitimacy of the judicial system is based on its status as a well-tested, publicly examinable, and heavily scrutinized tradition of practice. A shift to an unaccountable, opaque software system throws that basis away.
The problem of these feedback loops is similar to the philosophical problem raised in the film Minority Report, where a group of psychic precogs possess knowledge of future crimes, which the system then moves to prevent before they even happen. The question of how something could be predicted, if the prediction itself is used to alter the outcome, is also inescapable in machine learning.
In other words, there’s yet another problem: feedback loops, where usage of the system corrupts its own future training data. There can be further, more complex feedback loops. For example, if giving a harsher sentence for drug use increases the risk of re-offending by exposing an otherwise non-violent person to prison culture, then the machine system will continuously predict a higher and higher risk of re-offending with each re-trained version.
Major tech companies that need to make decisions about website optimization can deal with feedback loops in a variety of ways, such as randomizing certain application features, or leaving some users out of the system for better comparison of results. These types of solutions would correspond to something like randomly assigning a score to some people to gather data. This is, obviously, unworkable from a justice perspective. Many people might naively assume that a machine learning system would simply get better over time with just “more data,” but the presence of these feedback loops means that this is not the case.
So even working accurately, predictive statistical systems applied to the judicial system have many deep pitfalls in how they corrupt the incentives and stability that the system exists to produce. A bit of forethought shows that these limitations will be extensive: nowhere in the core purpose of the judicial system—to accurately punish committed crimes to prevent future crime via incentives and incarceration—is there a need for prediction, statistical or otherwise. Mostly, the judicial system needs to interpret qualitative evidence to decide what happened, whether that constitutes a crime, and if so, how severe of a crime. Machine learning and other statistical methods do not address these needs, so they are limited to secondary concerns, like flight risk and recidivism. Machine learning to predict crime may have its place, but that place is mostly not inside the judicial system per se. It may help in policing.
This bring us to the other flaw of these systems. They are just not that accurate. A group of Mechanical Turk participants was able to predict recidivism rate at a very slightly better rate than COMPAS for a data set of 1,000 criminals in Broward County, Florida. There are a few issues with the analysis in that study, but the general conclusion is startling for people with a lack of ML experience: a group of mostly random people, slightly filtered to make sure they can pass a basic reading comprehension test and given an extra $5 if their accuracy exceeds 65%, as a group, can have a prediction accuracy competitive with COMPAS, even though they had access to just seven features, instead of 137. This points to the idea that the system is not that good in the first place and/or that the “signal” is simply not there.
It’s unclear whether the “noise” present in the data is due to some sort of artifact of high-risk people not committing crimes because they are in prison, or some other feedback loop. Note that it’s not that random humans are particularly well-suited to the task, either. A simple linear classifier, or an even simpler algorithm that sorts people into high risk if they have three or more prior convictions and low risk if they have fewer, performed about as well as the complex system. To be fair to the system, COMPAS gives a 1-10 score, which the researchers compressed into 4 or less = low risk and 5 or more = high risk. The comparisons above are a binary classification. Having a more fine-grained classification is a plus, but for the task of predicting crime after two years, the system seems neither very accurate, nor beneficial from the perspective of an incentive structure, nor robust with regards to real-world complexities. Any one of these issues is a deal-breaker.
There is, of course, a more important question of whether these systems would reduce crime in practice. While it’s easy to assume that accuracy on a sub-task is correlated with improvement on a larger task of reducing crime, this is only true if the system is problem-free. And it isn’t. A tech company with skin in the game would not trust an improvement in prediction score alone and would A/B test the change to figure out if it makes money or not. It’s a little hard to come by or set up controlled experiments that compare districts where the COMPAS system was used or not used. Those studies would be the final arbiter of whether the overall system is working, rather than whether a sub-component has high accuracy. But note again the difference between reducing crime in the short term, and achieving the aims of the judicial system, including crime reduction, in the long term.
So far, we’ve discussed whether ML can productively predict the probability of a person committing an additional crime within two years. Other tasks in the judicial system are potentially easier to solve.
An interesting study has suggested that for the slightly different, secondary task of predicting flight risk or failure to appear in court, ML may work much better. In this case, the task is to determine whether a person will fail to appear in court, or will commit additional crimes while awaiting a court date.
The study showed that a custom-made ML system outperforms judges’ implicit predictions based on either reducing crime further, or keeping the amount of crime the same and reducing the number of people behind bars. The authors considered several alternative hypotheses, such as lenient judges being better at risk assessment than non-lenient ones, judges being constrained by variables such as jail capacity, and whether judges are trying to maintain racial equality at the expense of keeping high risk criminals jailed. They rejected all the alternative explanations. Instead, the judges primarily struggled with high risk suspects. Judges had an easy time determining that low risk suspects were, in fact, low risk, with high agreement between themselves and the algorithm. However, the algorithm seemed to outperform the average judge on predicting the highest risk cases. Even an algorithm trained to predict some individual judges’ scores itself performs better than the average judge. This suggests judges are failing to predict high risk cases in some unprincipled way, inconsistent with how they otherwise predict.
The study, despite otherwise being high quality, failed to answer important incentive and judge-training questions, such as: what is the discrepancy in accuracy between judges, and do they get better over time? Are high risk suspects being let out because the judge thinks they are not, in fact, guilty of the crime they were charged with? What are the key predictive variables that ML considers but judges do not?
This is a surprising discrepancy between two seemingly related tasks. On the one hand, we see evidence that a ML model can potentially make better predictions than judges about whether a person commits a crime before the next court appearance. However, the COMPAS model performs about the same as random people in predicting whether a person commits a crime within two years after sentencing.
So, for some sub-tasks of the system, there is potential for improving on judicial opinions, but this is still insufficient to declare human judgment flawed. It’s not even sufficient to suggest using ML as an input to the decision at all. First and foremost, it’s worth exploring more basic alternatives further, such as judge education in better risk assessment, as informed by the common patterns that the model discovers.
Both models can suffer from problems of destroying their own training data if deployed for an extended period of time (a variant of Goodhart’s law), and not being incentive-compatible with punishing features correlated with crime, instead of crime as such. If anything, this shows the potential for ML models to be better used in studying the judicial system and pointing out areas of improvement or further investigation. Instead of trying to shove ML into the decision-making process and forcing an explanation out of it, it’s worth studying the patterns that could be improved with better human decision-making. For example, it would be good to understand what the main features are that make someone both “high risk,” according to the ML system, and likely to be missed by judges in determining pre-trial crime rate. A broader knowledge of those features could be a useful guide, rather than being cloaked by opaque algorithms. The broader knowledge of crime and re-crime patterns can also inform broader policy questions outside of the judicial system.
***
A point sometimes made about ML technologies is that they are biased against certain ethnic groups. The focus on bias is unfortunate because it somewhat misses the point about broader incentives. But it’s still important to address, because allegations of bias are so prevalent. The metrics frequently used in bias discussions are deeply counterintuitive and unstandardized. To get a better sense of whether these metrics can prove bias or not, it’s worth using them to score less complex algorithms and see what they report.
An important sign that the metrics of bias themselves are flawed comes from the same study comparing the accuracy of random people and COMPAS. The authors declared the process their participants used to be “biased based on race.” This is rather curious because, like COMPAS, race is not under consideration. The study setup used the following question:
The defendant is a [SEX] aged [AGE]. They have been charged with: [CRIME CHARGE]. This crime is classified as a [CRIMINAL DEGREE]. They have been convicted of [NON-JUVENILE PRIOR COUNT] prior crimes. They have [JUVENILE- FELONY COUNT] juvenile felony charges and [JUVENILE-MISDEMEANOR COUNT] juvenile misdemeanor charges on their record.
Participants put a 1 or a 0 based on this description to predict whether the person re-offends within two years. Race never explicitly enters the equation.
More specifically, the allegation is the following:
Our participants’ false-positive rate for black defendants is 37.1% compared with 27.2% for white defendants. Our participants’ false-negative rate for black defendants is 29.2% compared with 40.3% for white defendants.
To see how this could happen, let’s subdivide the group of criminals in this data set by the number of prior convictions. We get the following differences in the subgroups, according to false positive/false negative rates:
priors>8 | FP rate | FN rate | |
White | 100.0% | 0.0% | |
Black | 100.0% | 0.0% | |
priors=3-7 | FP rate | FN rate | |
White | 88.9% | 2.4% | |
Black | 94.2% | 2.3% | |
priors=1-2 | FP rate | FN rate | |
White | 38.5% | 54.5% | |
Black | 28.6% | 43.3% | |
priors=0 | FP rate | FN rate | |
White | 5.2% | 97.6% | |
Black | 3.2% | 98.3% |
Note that the bias on race either shrinks, disappears, or even reverses when we look at subgroups with a different number of priors. This is a nearly textbook example of Simpson’s Paradox.
To explain the same data in another way, let’s say we only had the data of whether or not the person has <3 priors, or whether the person had >=3 priors, and we wanted to classify them as high risk or low risk. People with >=3 priors commit crimes at 60% rate, people with <3 priors commit crimes at 30% rate. If we move the >=3 priors to the high risk category and <3 priors to low risk, then we have a large false positive rate (in this case 100%) for the high priors group and have a large false negative rate (once again 100%) for the low risk group. Since race is correlated with priors, this will result in false positive/false negative rate differences for different races. As it turns out, black defendants come in with more priors on average than white defendants, and so are more often classified as high risk. A higher risk classification necessitates a higher false negative rate when a crime is decided to not have been committed.
People may see this as a situation of the bias disappearing, when controlling for prior convictions. However, the point is that the assessments of bias used by this study are going to label almost every algorithm as biased and thus are not only not useful whatsoever, but are actively harmful in understanding the functioning of said systems.
We don’t consider a system to be biased when two races commit different crimes on average and receive different sentences. However, risk assessment by any non-random binary classifiers would label risky criminals riskier than they actually are and label less risky criminals as less risky than they actually are. Note that COMPAS itself is not a binary classifier. It gives people scores from 1–10, which is more fine-grained than the compression of the data into high and low risk done by “journalistic analysis.” Still, it will have similar problems in practice.
In COMPAS, other factors besides prior convictions affect high risk vs. low risk judgments. People who commit felonies are higher risk than people who commit misdemeanors. People who are younger are at a higher risk. Black defendants commit felonies at a higher rate, are generally younger, and are generally more likely to be men. All of these factors are far more likely explanations for the false positive/negative rate discrepancy than any sort of bias. It’s not enough to just control for a few variables and call it a day. Claiming bias from different false negative/positive rates is about as honest as claiming bias because different races commit different amounts of crime and thus are scored differently.
To hammer down the point of why alleged false positive/false negative rates are extremely misleading, let’s look at the most basic raw data that generate them from ProPublica’s extensive 2016 investigation:
|
|
Note that despite a higher false positive rate, within the high risk group, black defendants have a higher recidivism rate (63%) than whites (59%). This shows that the system is not classifying more blacks as high risk than is warranted. If it were, there would be an anomalously lower re-offending rate.
It’s not just that ProPublica missed this metric. In fact, it used this “within high risk” rate metric for another pair of groups—men vs. women, to suggest bias against women.
The COMPAS system unevenly predicts recidivism between genders. According to Kaplan-Meier estimates, women rated high risk recidivated at a rate of 47.5 percent during two years after they were scored. But men rated high risk recidivated at a much higher rate—61.2 percent—over the same time period. This means that a high risk woman has a much lower risk of recidivating than a high risk man, a fact that may be overlooked by law enforcement officials interpreting the score.
That’s right. The analysis used two contradictory bias metrics, which point in the opposite directions. Using ProPublica’s own graphs:
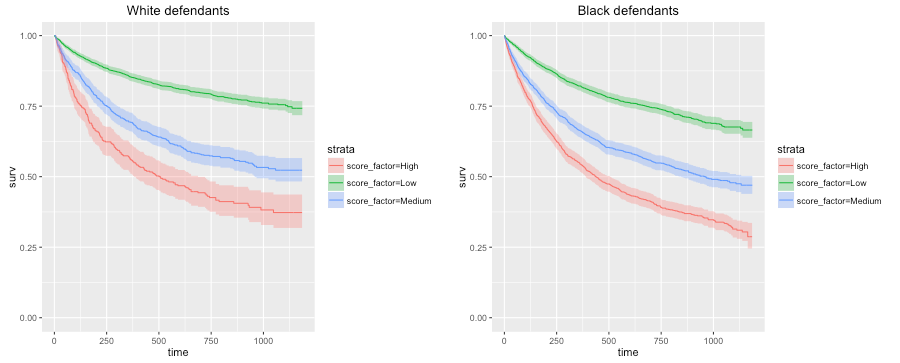
Corporate wants you to find a difference between the top and bottom.
The commonly cited bias statistics are misleading:
- They prove too much—proving almost every non-random algorithm is biased, no matter how simple.
- They disappear or diminish when controlling for prior convictions and other variables.
- The same data contradict intuitive notions of bias, namely that bias should result in lower recidivism in high risk cohorts.
- The metrics were dropped when inconvenient. This is not a statistical reason, but is suspicious.
Any of these is a potential deal-breaker.
There are some people who might be very excited to learn that the systems aren’t racially biased, and use this point as an argument for applying these systems enthusiastically, because they believe statistical systems can eradicate “judge bias.” A lot of confusion here is due to a frustrating misunderstanding of “cognitive bias” literature.
Like all humans, judges have “cognitive biases.” They can’t really intuitively do Bayesian probability puzzles without prior training, or they otherwise suffer from a number of known fallacies like confirmation bias, or the conjunction fallacy. While this is unfortunate, there isn’t as much evidence that those “divergences from Bayesian reasoning” somehow also lead to evaluating different races differently.
However, journalists like to use the word “bias,” which implies a relationship between cognitive and group biases, without evidence that such a relationship exists. Of course, a completely separate category of things we call biases, such as “political biases” obviously do affect decision-making, as evidenced by how frequently the Supreme Court splits based on political affiliation. There are also other examples of apparent bias, which are generally fake. A commonly cited proof of bias is that judges change their judgments based on how close lunch is. As it turns out, it’s far more likely to be a situation of judges taking up easier cases before lunch.
Machine learning is a powerful tool, but it’s difficult, and fundamentally suspect, to apply it directly in the process of the judicial system. Much more promising is using it as an investigative tool to find surprising statistical effects, like judges being too soft on specifically high-risk cases, which can then be tackled by more focused qualitative attention.
A common narrative in this subject is a kind of breathless millenarianism, where we’re about to replace obsolete human judicial systems with sleek AI machines and usher in a cyberpunk future of hyperefficient but morally challenging algorithmic crime prevention. But the reality is more boring. Machine learning in the judicial system is mostly over-hyped statistical tricks misapplied to secondary problems that are only partially about predictive accuracy. Even there, it barely manages to keep up in predictive accuracy with simple decision rules and Mechanical Turk workers.
The current trend in discourse is to chase around and try to correct non-existent biases. But this just corrupts and distracts from more important fundamentals. The mature human social technologies we rely on, like our judicial tradition, are far stronger and more nuanced than is usually assumed in idealist and futurist discussions, and can’t just be automated away, for either bias or efficiency reasons.
The downsides of machine learning in the judicial system, in self-corrupting feedback loops, bad incentives, lack of performance, opacity, and sheer inappropriateness for the problem at hand would seem decisive, but the hype marches on.